Biometric systems are becoming increasingly prevalent in today's interconnected world. These technologies compare biometric data provided during verification with a previously stored template to allow authentication or identification of the person. They represent a unique innovation capable of simultaneously enhancing security and user experience. This makes biometric systems an attractive choice for key applications such as Remote Identity Proofing solutions, secure access control with passport eGates or unlocking personal devices as smartphones.
However, the speed at which the ecosystem has grown has brought challenges. The fragmented development of various components, coupled with the limited sharing of information about the internal mechanisms of systems, has meant that in certain scenarios, the performance of some solutions is limited by a notable issue: biases. If left unchecked, biases can result in differential performances and security issues for some demographics groups when deployed in real life.
Bias in biometrics.
The algorithms and software that power biometric systems are built using artificial intelligence (AI). For example, most facial recognition systems are built on Convolutional Neural Networks (CNNs) such as ArcFace architecture, which allows for deep machine learning based on the templates presented. There is now consensus that an AI model is only as good as the data that trained it. With biometrics, algorithms are trained using genuine and spoofed image datasets. If the data is not diverse then the algorithm’s performance will be inadequate. This is bias. It is not necessarily malicious, but it is a reality.
Such biases, if not addressed, can lead to unequal performance across demographic groups. If a certain group has a poorer experience due to their biological characteristics this might result in lower adoption, and a facial recognition technology that cannot perceive between individuals of any given demographic presents a security risk. Not only does lower adoption risk the financial viability of a product, it risks reputational damage if a product is perceived to have a racist, sexist, ageist or other bias.
This needs urgent attention across the biometric value chain; from software and sensor developers to device OEMs and standardization bodies and governments.
Test bias to address bias.
The performance of a biometric system is evaluated against two primary parameters; False Accept Rate (FAR) is where impostors are mistakenly accepted as genuine users; and False Reject Rate (FRR) is where genuine users are wrongly denied. The challenge for biometric system providers is to keep these rates as low as possible for all demographic groups, ensuring inclusive biometric solution with high security and convenience for all.
Biases in these systems can be detected by the disparities in FAR and FRR when evaluating different demographic groups. This bias has been traditionally measured by biometric fairness metrics such as Fairness Discrepancy Rate (FDR), Inequality Rate (IR), Gini Aggregation Rate for Biometric Equitability (GARBE) or Separation Fairness Index (SFI).
To know the best metric for evaluating the potential bias of a biometric system, it is important to measure the effectiveness of each metric on the specificity and accuracy of the system. With this in mind, leading biometrics experts have created an innovative method to inject bias on biometric systems and evaluate the effectiveness of each metric. This system allows experts to inject selective biases for specific demographic sub-groups, with control over the strength of each bias. Exercising direct control over the strength of each bias allowed the expert to monitor the effectiveness of each metric against known variables.
Each sample was tested under two distinct testing scenarios; one with no modifications to the presented sample, and one with a progressively modified variant on a specific demographic characteristic to cause a variation in performance and create bias. This variation of the demographic characteristic allowed the experts to measure the sensitivity of the metric to each bias; if the value of a metric correlates with the change in bias, it indicates that the metric could be used to investigate bias in the industry.
Key findings.
Once the fairness metrics on the data sets for both the unbiased and the synthetically biased scenarios had been computed, using the Pearson Correlation Coefficient allowed the experts to visualize the linear relationships between the metrics and the bias introduced. They could then compare how the fairness metrics responded to each of the synthetic alterations. Metrics controlled by an alpha parameter – a variable value used in FDR, IR, and GARBE to achieve equity between security and the user experience – are less stable than those without one.
The findings propose a new fairness index in the form of the Area Max Differential Rate (AMDR), which identifies the differential between False Match Rate and False Non-Match Rate as the hallmark of an unfair system and does not rely on an alpha parameter. This is better suited for detecting variations across the three types of systems with different loss functions. Each system manufacturer uses its own model based on a specific type of loss function, so identifying the most appropriate metric, or combination of metrics, to apply in each specific case is a significant step toward achieving fairness.
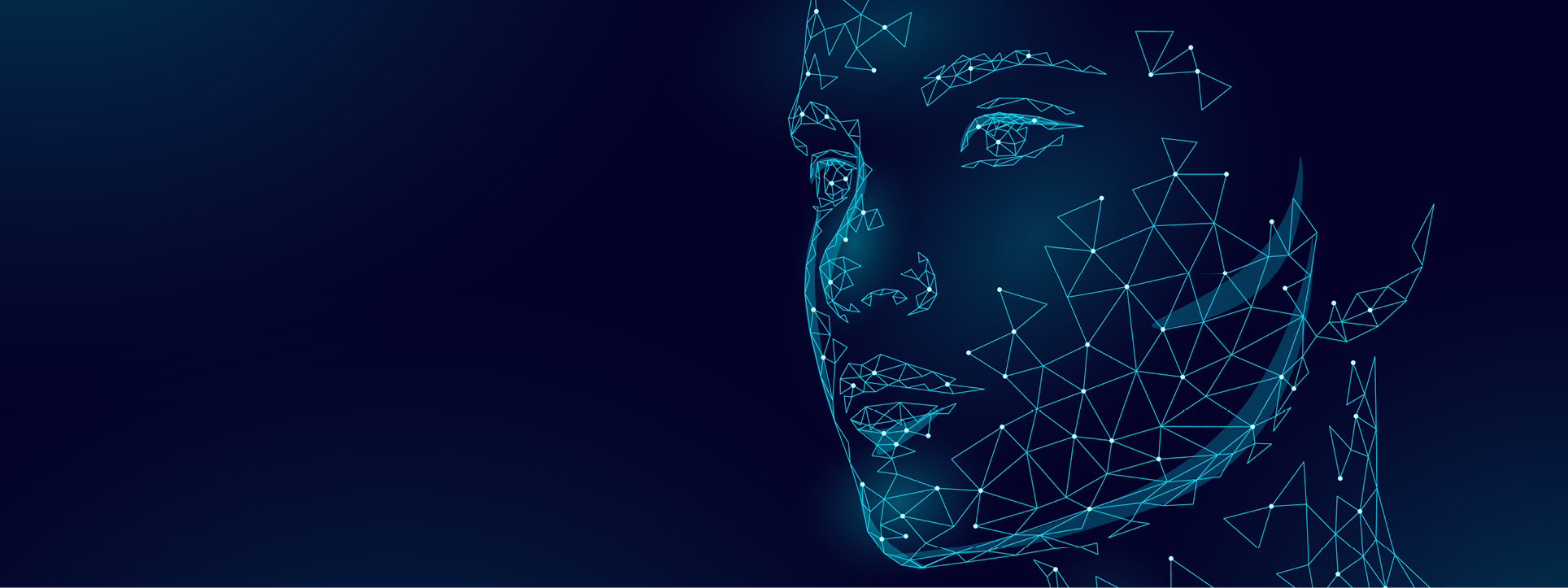
Raising the bar for biometrics.
This examination of bias in facial recognition systems underscores the need for stringent requirements regarding the accuracy and fairness of all biometric solutions. It demonstrates that, while biometric systems have developed rapidly over the past decade, work is still to be done to enhance their security, practicality, and inclusivity.
Introducing new methodologies to evaluate the effectiveness of bias detection with different metrics, allows vendors to better train their solutions to account for biases and elevate real-world solutions. In doing so, solution providers can enhance their offer to ensure that both they and the OEMs that use their products do not jeopardise user data or risk the reputational damage of uncontrolled bias. Standardization and certification bodies can also use this research to augment requirements, standards and test plans.
This article was first published by Biometrics Institute and is reproduced with permission.