Joël Di Manno, Jean Fang and Abdarahmane Wone discuss addressing biometric spoofing using synthetic data in this article for Biometric Technology Today.
The rapid growth in the Internet of Things (IoT) and connectivity is transforming industry sectors that previously have been left untouched by the world of connected devices. In areas ranging from large-scale infrastructure projects down to fish tank temperature sensors (1), linked devices are now ubiquitous - and each and every one of them is at risk of attack.
Personal authentication in this increasingly connected world is especially vulnerable: if fraudsters are able to falsify people’s credentials, they can access potentially critical data across ecosystems including payments, access control and healthcare.
Clearly, biometric checks enable individuals to authenticate themselves in both a more convenient and robust way, compared to conventional techniques like weak and inconvenient PINs and passwords. Physiological and behavioural characteristics like the user’s face, iris, fingerprint, voice or how they type their password offer a convenient and secure way for people to authenticate themselves when using a device - so it’s not surprising that they are already being used so widely and adoption continues to grow.
Within this, fingerprint sensors remain one of the
most common biometric deployments. They offer a cost-effective and easy-to-implement
solution that guarantees high identification accuracy, which is why we have
seen them utilised in applications ranging from approving banking transactions
to unlocking a phone. However, this universality also makes them a prime target
for fraudsters to attack.
How fingerprint spoofing works.
Presentation attacks such as spoofing can compromise fingerprint biometric authentication. These attacks occur when artificial prints made from simple household items including gelatin, wood glue or latex are misused to clone fingerprints, in order to carry out identity and access fraud.
To counter this, it is imperative that the presentation attack detection (PAD) technology in a biometric solution is thoroughly tested, as this is the core means to ensuring the security of the system.
PAD testing is usually done by creating ‘presentation attack instruments’ (PAIs) and using these perform active spoof attempts - to determine whether a biometric system will authenticate a credential that is not genuine.
However, replicating all the possible options that fraudsters could use to execute an attack requires a significant investment from labs. The vast number of household items that are readily available and could be used to create a cloned fingerprint means that a large volume of testing must be done. The lab must also have the skill to create each of these different types of spoof to the high standard that fraudsters are able to achieve.
Doing this extensive amount of work manually can also incur high overall costs for each novel material and fingerprint sample involved. So this combination of factors forces certification bodies to make choices that may limit the number of combinations they test, to keep control over the cost and time spent on each session.
Advances in the field of fingerprint spoof detection have also been inhibited by the lack of large-scale and publicly available fingerprint datasets. And with data protection regulations becoming increasingly stringent to address concerns around personal privacy, sharing these fingerprint collections across organisations is increasingly challenging.
This is greatly limiting further developments in anti-spoofing AI, as most state-of-the-art algorithms use deep learning architectures to build a cache of information that can be used to enhance and refine each algorithm.
If such systems do not have the multiple layers of data they need, they are not able to accurately extrapolate previous results into new contexts. This means that the algorithm is not able to optimise and refine its simulations as accurately.
In summary, there is a limit to the range of different
spoof species and spoof quantities that can be used to effectively test a
solution when using physical creation methods.
Synthetic spoofing.
To solve this problem, artificial Intelligence can be used to mimic part of the spoof in a hybrid evaluation process (physical and simulated) in order to help cover a much larger number of spoof cases. And synthetic data offers a way for labs to resolve many of the issues that they face with current manual spoof testing processes.
So what does this involve? The first step towards effective synthetic fingerprint spoof testing is to generate a large enough database.
A select number of public datasets currently exist, such as the Fingerprint Liveness Detection Competition LivDet. This is an international dataset consisting of both live and fake images that utilise a range of different common spoofing materials. Homemade databases can also be useful when preparing an AI algorithm to simulate a new spoof species.
New solutions based on deep learning can use these genuine fingerprint images and transform them into what they would look like if they were created from the materials that are generally involved in fingerprint spoofing tests (such as wood glue, latex, gelatin, Ecoflex, etc).
Digitally synthetised fingerprint spoofs (DSFSs) help to cover a larger number of spoofs materials than it would be possible to physically fabricate in a given time.
Using this method, the testing process can be both speeded up and improved. Not only does this reduce the time and cost investment required by labs, it also gives the deep learning algorithms enough data to produce even more accurate simulations.
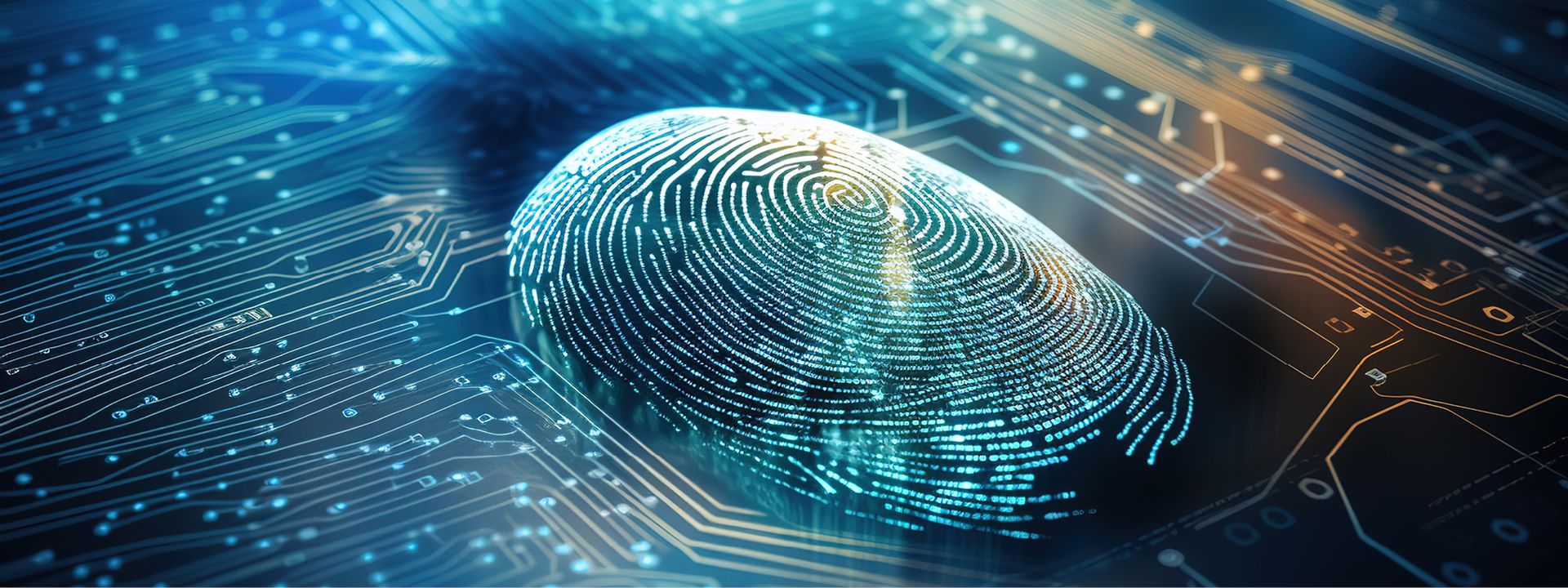
Building trust in the synthetic approach.
Biometric authentication can provide robust security and a frictionless user experience - if it is implemented correctly. Testing and certification are therefore fundamental to supporting the continued evolution of biometric identification.
Robust testing and certification protocols ensure that any product meets the latest protections benchmarked against best-in-class solutions. In this way, synthetic data is helping to enhance certification programmes – and by extension to combat fingerprint spoofing attacks – by allowing test protocols to evaluate a much wider range of spoofs at lower costs.
By using hybrid evaluation methods, certification can lead to more accurate, trusted biometric PAD systems with an optimised IAPAR (impostor attack presentation accept rate), APCER (attack presentation classification error rate), and BPCER (bona-fide presentation classification error rate).
Algorithm providers also benefit from the use of synthetic data. Often they do not have the expertise or test tools needed to give their algorithm the information it needs to learn. By using AI-based synthetic spoofing, these providers can quickly simulate hundreds of samples, thereby allowing them to further refine their solution and build trust in anti-spoofing technology.
Environmental impacts.
Ensuring biometric performance across varying environmental conditions is another challenge that synthetic data can help to resolve.
Fingerprint systems can be affected when environmental conditions like temperature and humidity change: the texture of a fingerprint alters accordingly, but this change may not be accounted for by the sensor. This difference can lead to false rejections, as the valid print being used does not match the template fingerprint recorded during enrolment.
Using synthetic data, enhanced biometric solutions can be developed that account for the changes to an individual’s fingerprint caused by different environmental factors. In this way, a digital print can be artificially replicated across a host of different weather conditions to ensure that the same fingerprint is recognised regardless of humidity or temperature, but without compromising on security.
By making use of synthetic spoofs and AI, evaluation can be done efficiently without the need for a climatic chamber, saving time and money.
In short, testing using synthetic data allows solution providers to further develop their algorithm to ensure that the product can be deployed globally and will perform well in different climates. By taking these factors into account, they can enhance the trust, security, performance and user experience of their solutions.
Research findings.
Fime has researched the impact that digitally synthesised fingerprint spoofs can have on anti-spoofing systems, in collaboration with Normandie University, UNICAEN, ENSICAEN, CNRS and GREYC (2).
This study focused on determining whether digitally synthesised images are as good as real spoofs. The research team used AI and deep learning to transform genuine fingerprint images into spoof images similar to the ones made from the spoof materials commonly used in anti-spoofing tests. We did this in order to simulate the standard testing process.
We used a multi-domain style transfer model taking data from LivDet, an international fingerprint liveness detection competition that brings together academies and private companies that deal with the problem of presentation attack detection. Data from five different materials were used: Ecoflex, gelatin, latex, modasil and wood glue.
The dataset was composed of a training set and a testing set, each containing 2,000 images (1,000 genuine images and 200 of each spoof material for each set). We extracted and randomly cropped multiple 224 x 224 patches from each image and injected them into the system to see if they were detected as spoofs under the NIST Fingerprint Image Quality (NFIQ) algorithm.
To assess the validity of the digitally synthesised fingerprint spoofs, the NIST Fingerprint Image Quality (NFIQ) algorithm - which provides an overall score on a scale of 0 to 100 - was used. This is based on the usability and features of an image.
We used this algorithm to determine whether the quality of the presentation attack instruments was similar to that of the synthetic presentation attack images. And for each material, we found that there is a similarity between the distribution of the genuine images and synthetic images.
Based on this, Fime has developed a method that can be used to evaluate the ability of biometric systems to resist fingerprint spoofs. This can help vendors to develop their fingerprint recognition products, in particular training algorithms to resist presentation attacks.
These findings will ultimately help laboratories to make cost and time savings, helping secure products launch more efficiently.
Future of biometrics.
In conclusion, as AI and deep learning systems continue to evolve, we will be able to do even more to improve biometric authentication.
We have seen that labs will be able to continue improving how they evaluate biometric systems’ resistance to spoofing. Vendors can further enhance their biometric products; and certification bodies, for example, could use the research to implement new testing methodologies for these products.
These findings will ultimately help laboratories, certification bodies and biometric solution providers to make cost and time savings, and help to ensure that secure products are launched more efficiently.
Yet despite the clear utility of synthetic data in combatting fingerprint spoofing, several limitations remain.
For example, work is underway to enhance the diversity of the generated images. This diversity is essential to the future of synthetic spoofing, by providing the same level of functionality and security against attack regardless of the age, gender or race of the reference fingerprint used.
Synthetic spoofs must also be able to reproduce the range in quality of physically created spoof samples. In addition, the number of novel spoof types continues to grow, and meeting the challenges presented by each of these will require continued innovation and investment.
But while these innovations may still be in
development, as more work is done to enhance synthetic algorithms, fingerprint
sensor deployments will continue to grow.
References
1. Alex Schiffer. ‘How a fish tank helped hack a casino’. Washington Post, 21 July 2017. https://www.washingtonpost.com/news/innovations/wp/2017/07/21/how-a-fish-tank-helped-hack-a-casino/.
2. Fime. ‘Digitally synthetized fingerprint spoofs: a threat for anti-spoofing systems?’. https://www.fime.com/blog/scientific-papers-27/post/digitally-synthetized-fingerprint-spoofs-a-threat-for-anti-spoofing-systems-414?utm_source=webpage&utm_medium=blog+antispoofing&utm_campaign=scientific+paper.
Read other blogs in our biometrics series: