Increasingly, strong security measures are required to protect valuable data from fraudsters. However, as security measures increase in complexity, the process becomes more cumbersome for consumers.
Banks and vendors face the challenge of meeting security regulations, such as PSD2, while providing a seamless authentication journey for consumers. To meet PSD2’s Strong Customer Authentication regulation, multi-factor authentication can be used to provide additional layers of security. As part of this, biometric technology is frequently applied – with one such method being keystroke dynamics.
Stéphanie Pietri (SP), Communications Director at Fime, speaks to Yris Brice Wandji Piugie, Artificial Intelligence, Biometrics Researcher at Fime, about Fime’s new research paper to explore new ways to deploy keystroke dynamics to authenticate users. The paper was awarded best paper at the Cyberworlds 2022 event in September.
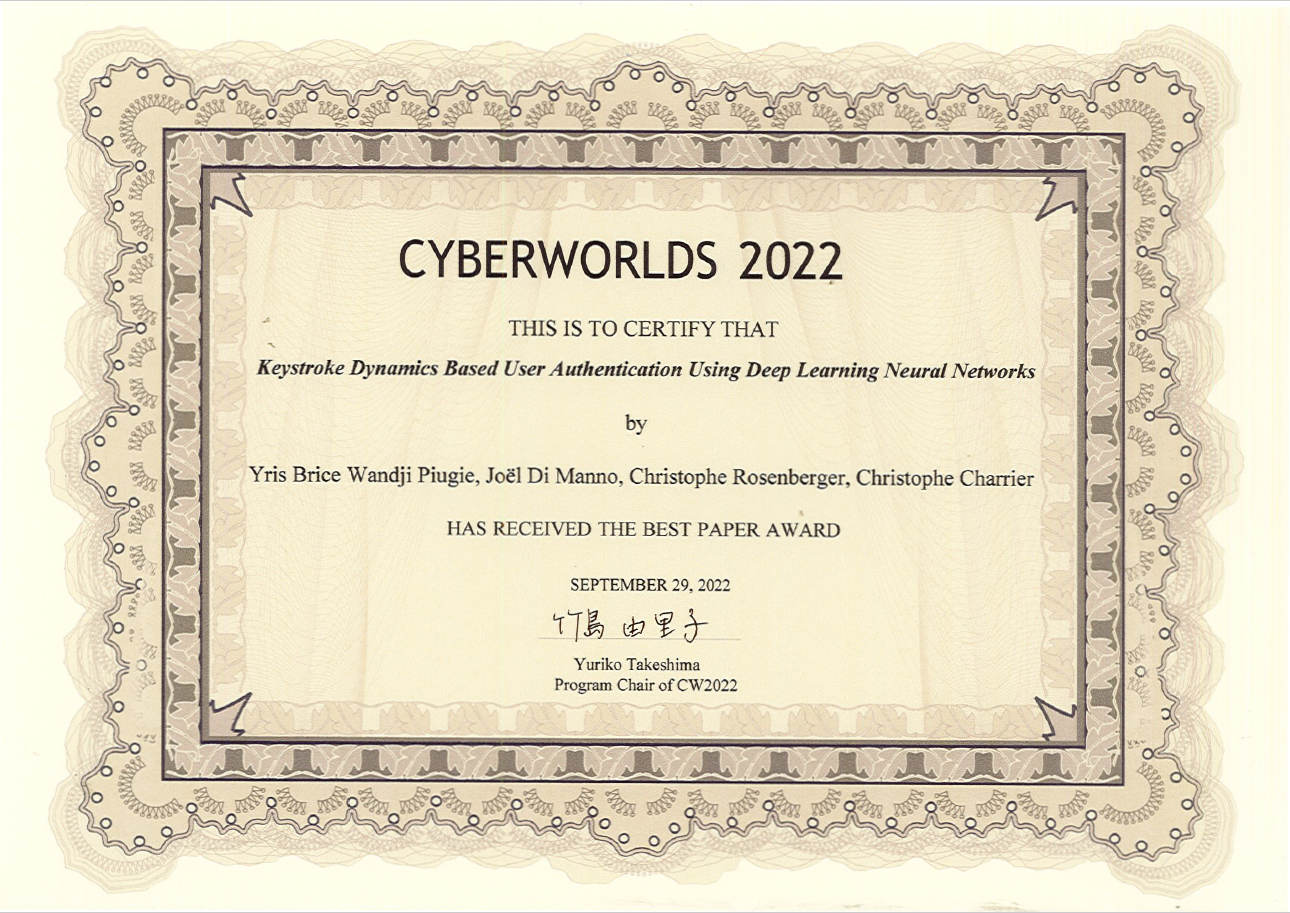
Stephanie Pietri: What is keystroke dynamics?
YBWP: Keystroke dynamics is a behavioral biometric modality that analyzes how a user types on a keyboard. It analyzes the manner and rhythm of the user’s typing before storing the data to be compared to future typing. Keystroke dynamics can be used as a multi-factor authenticator, as it combines the knowledge of a password and the manner of typing.
SP: What did Fime do?
YBWP: Fime has developed a new approach to using keystroke dynamics, introducing deep learning architectures. Deep learning is a machine learning technique which uses neural networks to analyze data and learn from examples.
Fime took keystroke dynamic data from 110 users who typed in the same password from the GREYC-NISLAB database. The data was converted into 3D images or 2D color images that could be used to represent a user’s behavioral typing style and compared with others. Fime then used a matching algorithm to determine how closely each biometric credential matched with an existing template in the database. Using 3D images or 2D color images to train the neural network, this algorithm was used to evaluate the degree of similarity between how the password was entered.
To measure the performance of a biometric authentication system, two important error rates are used: False Match Rate (FMR) and False Non-Match Rate (FNMR). The False Match Rate error measures the likelihood that the system will incorrectly match a person to someone that is enrolled in the system. The False Non-Match Rate error measures the likelihood of the system failing to recognise a person who has enrolled in the system. The Equal Error Rate (EER) is when the FMR is equal to the FNMR. We evaluated the performance of the authentication system in terms of Equal Error Rate (EER).
The lower the value of EER, the better the performance of the authentication system.
SP: What were the findings of this research?
YBWP: Overall, the research validates the use of keystroke dynamics for user authentication but highlights the need for a large data set.
We found that when we look at the performance per password (looking at each dataset individually), using different passwords generated different results. We also found that using a small number of samples (7) for a user led to poor results, with an EER value between 14 and 18%.
This is because deep learning techniques require a larger amount of data - the larger the dataset, the better the performance. This highlights the need for large keystroke dynamics datasets to optimize the performance of these deep learning methods. In future, having a larger database, we would expect to get even better results, for example with an EER value very close to 0%.
In addition, when comparing our research to other research conducted using the same GREYC-NISLAB database, we found that our approach performed better with a lower EER value.
SP: What are the benefits of this approach?
YBWP: The aim of this research was to evaluate the use of keystroke dynamics as a behavioral biometric, and how deep learning can be used to improve the reliability of this type of authentication. As the power of machine learning is harnessed, behavioral biometrics can become more accurate.
This approach reduces friction because it does not require current users to change their behavior, since they have to type passwords in anyway.
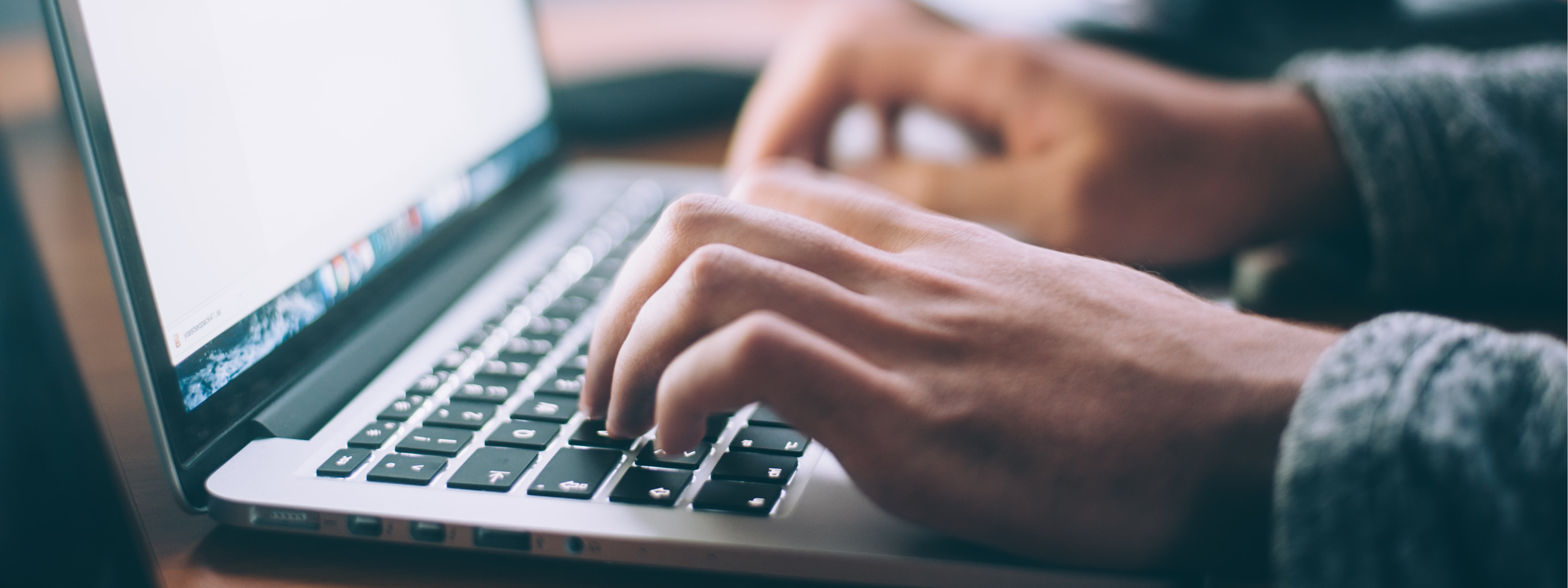
SP: What does this mean for the future of biometrics?
YBWP: This research is a positive step forward for us to better understand how keystroke dynamic-based authentication methods can be enhanced to deliver a seamless customer experience. While removing all passwords is something that we may see in the future, this is not expected anytime soon. Therefore, it makes great sense to harness the data available to increase security and reduce friction without changing consumer habits.
This research is also the first step in using behavioral biometrics, and we do not intend to stop here. In the future you could use behavioral biometrics such as stride length when walking, or the way someone moves, to authenticate them. As the user experience grows in importance, we can expect to see demand to increase for this type of authentication.
For future research, we plan to add psychological features such as the user’s emotions when entering passwords for the training and testing process to improve accuracy, since emotional states could be identified from the input behavioral style. We intend also to improve (and expand) the keystroke dynamics datasets that are needed to make further significant progress to solve this authentication challenge.
Fime is dedicated to continuing its research to improve the performance, reliability and strength of biometric authentication. As part of this, it aims to support the development of secure, seamless and inclusive biometric systems. To learn more about our research on this topic, read the full paper here.
You can also listen to the Fime podcast.
Read other blogs in our biometrics series: